By Vishal Goel, | December 20, 2016
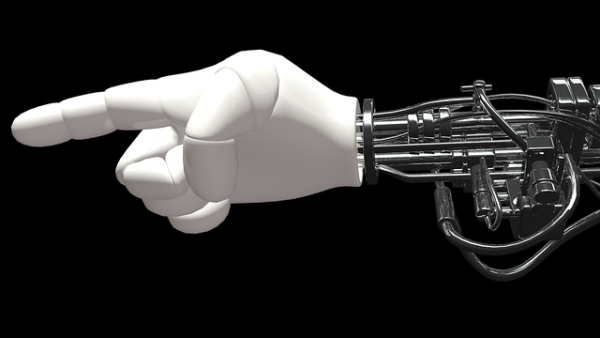
The test is aimed at machine learning programs, which learn to make predictions about the future by crunching through vast quantities of existing data. (Pixabay)
A team of researchers led by Google scientist Moritz Hardt has developed a test to detect any gender or racial bias introduced by Artificial Intelligence algorithms into the decision-making process. The approach is based on the concept that nothing about an individual's race or gender should be revealed by the decision, other than what can be extracted using input data.
Like Us on Facebook
Hardt, a co-author of the paper presented at the Neural Information Processing Systems (NIPS) conference in Barcelona this month, pointed out that decisions based on machine learning can be both incredibly useful and have a profound impact on our lives. Despite this, a vetted methodology in machine learning for preventing discrimination based on sensitive attributes has been lacking.
Since the decision-making criteria is essentially learned by the computer, rather than being pre-programmed by humans, it is difficult to know the exact logic behind decisions, even for the scientists who wrote the software.
"Even if we do have access to the innards of the algorithm, they are getting so complicated it's almost futile to get inside them," said Nathan Srebro, a computer scientist at the University of Chicago and a co-author of the paper. "The whole point of machine learning is to build magical black boxes."
To get around this, Srebro and his colleagues devised a way to test for discrimination simply by analyzing the data going into a program and the decisions coming out the other end. They called the approach Equality of Opportunity in Supervised Learning.
"Our criteria does not look at the innards of the learning algorithm," said Srebro. "It just looks at the predictions it makes." The approach has been explained with the help of a few examples as well.
While the US financial regulator, the Consumer Financial Protection Bureau, is interested in using the method to assess banks, others have raised concerns that the approach appears to ignore requirements for transparency about how decisions made by algorithms are reached.
-
Use of Coronavirus Pandemic Drones Raises Privacy Concerns: Drones Spread Fear, Local Officials Say
-
Coronavirus Hampers The Delivery Of Lockheed Martin F-35 Stealth Fighters For 2020
-
Instagram Speeds Up Plans to Add Account Memorialization Feature Due to COVID-19 Deaths
-
NASA: Perseverance Plans to Bring 'Mars Rock' to Earth in 2031
-
600 Dead And 3,000 In The Hospital as Iranians Believed Drinking High-Concentrations of Alcohol Can Cure The Coronavirus
-
600 Dead And 3,000 In The Hospital as Iranians Believed Drinking High-Concentrations of Alcohol Can Cure The Coronavirus
-
COVID-19: Doctors, Nurses Use Virtual Reality to Learn New Skills in Treating Coronavirus Patients